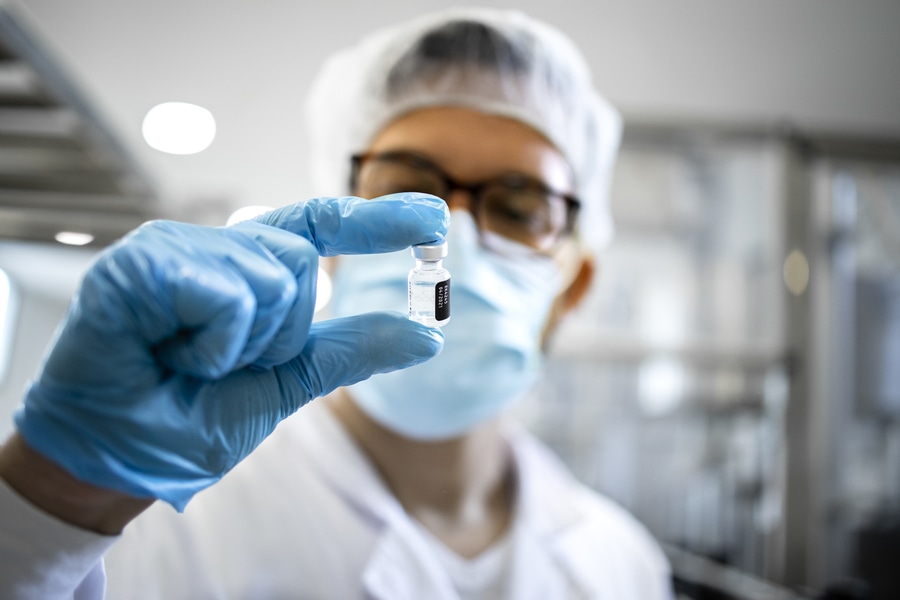
#Industry News
How Generative AI Makes Drug Discovery Faster and Cheaper
Generative AI is poised to revolutionize pharmaceutical drug discovery, empowering molecule discovery, antibody design, and even precision drug development.
It's impossible to escape the news about artificial intelligence and the impact it's having on almost every industry. From manufacturing, to banking, to healthcare, AI's ability to analyze vast amounts of data and develop new insights makes it extremely useful for any industry that must go through massive amounts of information. This includes the pharmaceutical industry and drug discovery.
There are an estimated ten to the sixtieth power number of drug-like molecules. Discovering, synthesizing, and testing such a massive number of potential molecules is obviously impossible. However, generative AI in drug discovery can search through vast swathes of data in seconds, creating new drugs that could otherwise take months or years.
How Generative AI Can Be Used in Drug Discovery
The typical drug discovery process can take over a decade, from initial concept to clinical trials and public release. This process also costs billions to complete, which means companies have every incentive to shorten this time and save money. For many, generative AI is the key to achieving this goal. AI is being used in applications such as:
Molecule Generation
Generative AI's ability to simulate molecular structures and how they interact with a patient's body can be used to develop molecules with desirable properties that are still safe for patients. By combining AI models with modern molecule development techniques, pharmaceutical companies can accelerate the process for creating new drugs.
Antibody Design
Generative AI can be trained on protein sequences and then used to create specific antibodies targeting pathogens. These protein language models can improve the quality and speed of antibody design and even create antibodies that are are entirely novel, meaning they do not rely on training data and can be tested and verified in a real-world lab.
Drug Repurposing
Drug repurposing lets pharmaceutical companies discover new uses fro drugs already approved for the public, letting them avoid the usual development costs and time investment associated with drug discovery. For example, the drug semaglutide was originally used to help diabetic patients manage their condition but was later adapted for weight loss as Ozempic. AI algorithms can quickly review existing scientific knowledge databases to explore new applications for pre-existing molecules.
De Novo Drug Design
AI models can even generate completely new molecular structures and predict how they interact with biological targets. This approach can be applied to atom-based, fragment-based, and reaction-based approaches for creating new structures, and lead to de novo drug designs that approach problems from a wider variety of angles.
Precision Drug Discovery
Precision drugs are highly desirable for healthcare, as they can help doctors treat a patient’s condition more accurately than a generic prescription. However, the process of creating custom medication for every individual patient is obviously impossible under the current paradigm. AI’s ability to analyze multimodal datasets such as patient data, genetic information, biobank studies, and more can help design drug candidates that are custom-tailored to a patient’s exact needs.
Challenges For AI in Drug Discovery
As with any new technology, there are challenges and hurdles to overcome for AI-powered drug discovery.
Generative AI can sometimes create "hallucinations," incorrect results that usually come about from insufficient data or inaccurate assumptions made by the AI. While this can sometimes be useful in drug discovery as it leads to new possibilities that researchers hadn't considered, it can also lead to the AI suggesting chemical compounds that are impossible to create in real life.
The solution for this problem is to train AI models on molecules and chemical reactions known to be valid, such as Stanford Medicine’s SyntheMol AI. This ensures that the AI only suggests drugs that can actually be created.
The second major issue is hardware for AI. Current AI models are heavily dependent on parallel processing, which allows them to analyze vast amounts of data simultaneously. However, parallel processing requires specialized computers with the right equipment, such as powerful medical panel PCs supported by the right processors and GPUs.
Final Thoughts
While they require effective training models and powerful hardware, AI-powered drug discovery promises to revolutionize the pharmaceutical industry with faster, cheaper development.
If your company is exploring generative AI and looking for a reliable hardware solution to support it, contact the team at Cybernet Manufacturing. Our experience as an original equipment manufacturer for a range of medical computers and tablets means we can design and build a device to your exact needs, letting you take full advantage of the latest in generative AI solutions.